Traditionally, companies use scheduled maintenance. A machine part that has performed its work for a set number of cycles is replaced. Predictive maintenance lets the machine part tell you when it needs to be changed. Using this simple shift of aspects you can reduce your downtime and optimise the periodic maintenance. Your finance department will thank you for not exchanging fully functional parts. And the environment will also thank you for reducing the need for resources.
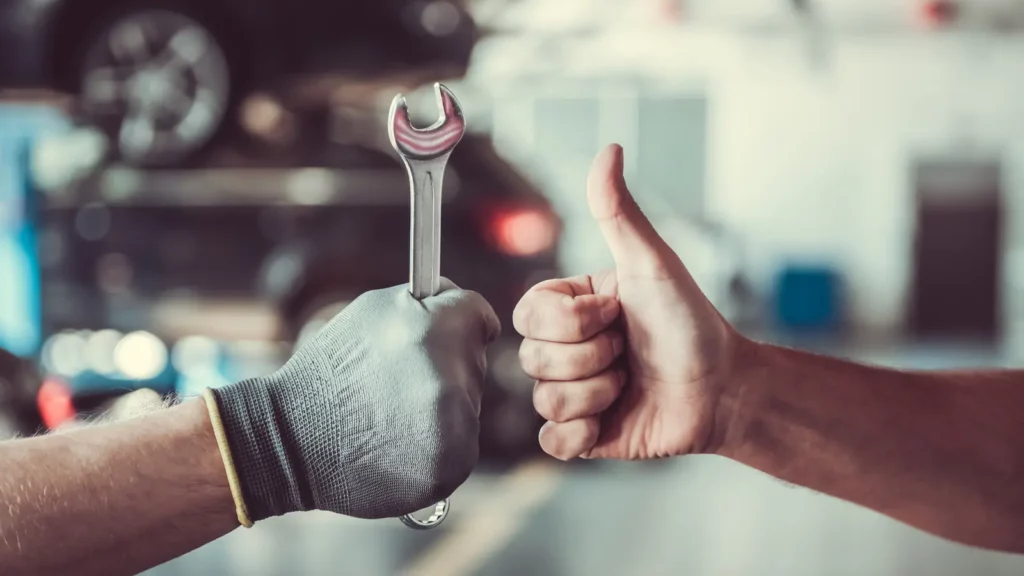
Edge Opportunities
Traditional predictive maintenance relies on models trained on significant amounts of data. Ideally, the data should include both positive and negative data points. That is to say, it should include data from well-functioning devices as well as failures.
To avoid the need of all that data, Ekkono’s SDK enables model building based on health indicators and incremental learning. The incremental approach builds upon the condition monitor use case. In predictive maintenance, we also start with a short initial training period to determine the normal state of the equipment. The normal state is then used for drawing conclusions about current and future maintenance needs. Working with predictive maintenance gives you the possibility to schedule maintenance well in time. This will make your technicians happier, and it will minimise downtime.
Predictive Maintenance
Problem
Time based maintenance results in a non-optimised maintenance of equipment. There is a risk of missing out on early indications of failure, leading to unscheduled downtime. There is also a risk of replacing fully functional machine parts.
Solution
Train a machine learning model to be able to predict future system health. Using incremental learning, the result is a personalised predictive maintenance system, tailored to the specific device.
Application
Apply conditions on the predicted future system health to determine when maintenance is required. If it is now or in the near future, trigger automated maintenance alerts.
Read more about our Use Case Archetypes